Realizing The Potential Of AI And Machine Learning In Clinical Research
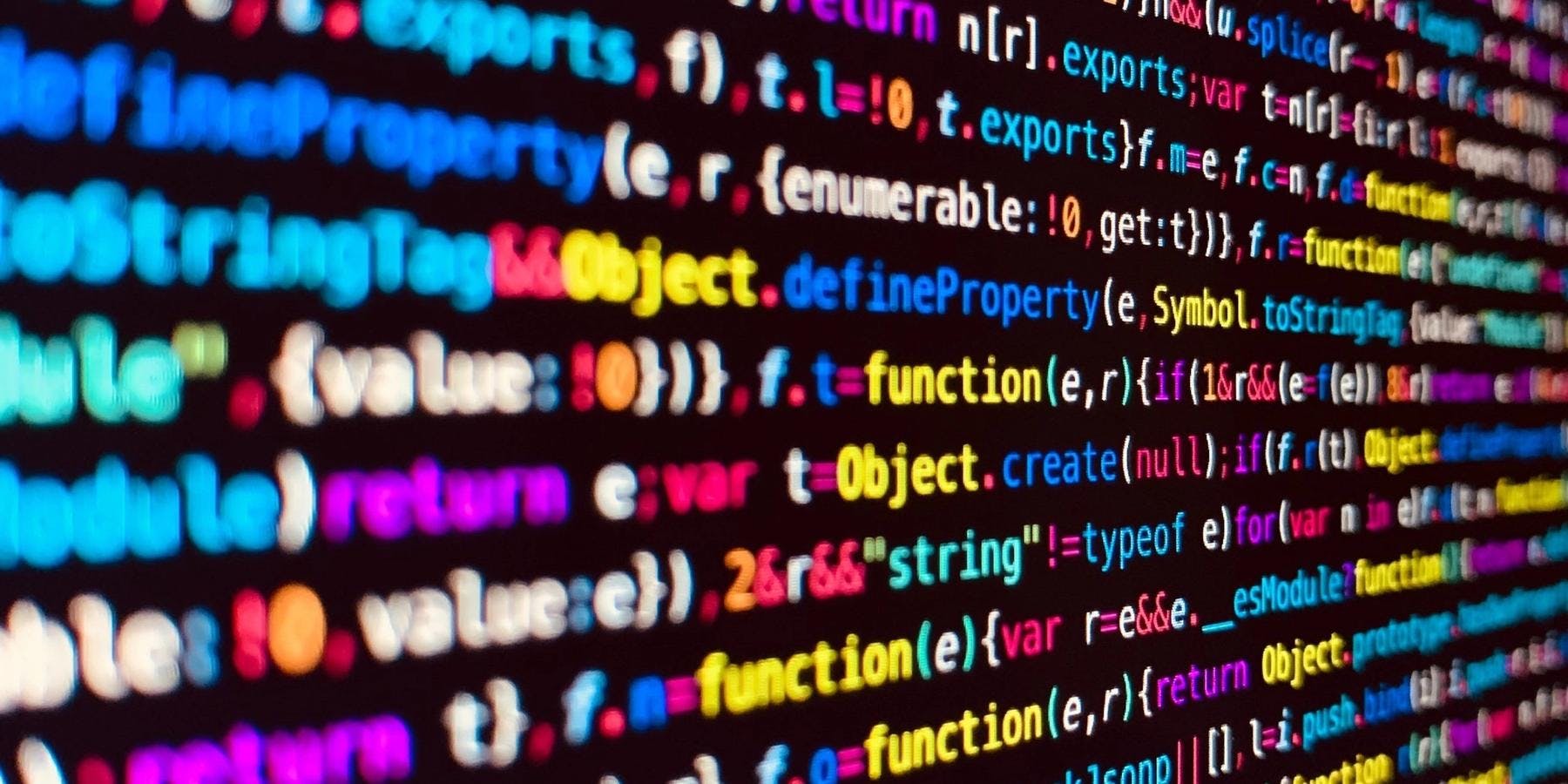
Over the last few years, talking about artificial intelligence (AI) and machine learning (ML) could drive customer interest, make something sound more innovative or even appear to boost a company’s valuation. Unfortunately, many of these AI/ML talking points were general, potentially premature and not yet ready to offer results. The clinical research industry was not immune to these discussions and may largely still view many AI and ML approaches as something on the horizon rather than as something available today.
In my time as a CEO in the clinical trial platform arena, I have seen how clinical research studies continue to become more complex, focus on more targeted disease areas and cost more than ever before. In parallel to this evolution, the clinical research industry has a goal of doing more—conducting more targeted studies, enrolling more global participants more inclusively, gathering more data, etc. Achieving these goals in the face of more complexity with less money and fewer people requires the industry to eliminate as many inefficiencies as possible.
AI and ML now have an opportunity to provide positive value and impact in our industry. Using them both allows us to automate common tasks, augment study teams to support action-oriented work, and provide advanced notifications to potential upcoming risks. Automating these tasks can also reduce time commitments, thereby providing clinicians more time to support and connect with their patients.
The life sciences industry has certainly not scaled or realized the full potential of AI/ML, but several use cases are available today to help start the journey. Here are four real-world use cases that can encourage researchers to unlock new efficiencies and insights in their studies.
1. Gathering, Analyzing And Implementing Participant Feedback
A powerful example of effectively using AI and machine learning is to bring the voice of the patient consistently into clinical research by listening to their feedback and analyzing responses throughout the clinical trial process. For example, AI is already helping to automate survey and voice data capture, minimizing the time needed for people to collect and analyze this data. As soon as the data is captured, AI is used to measure inflection, pitch and emotional cues that human observers may often not recognize.
The power of the AI and analytics processing is then augmented with human linguistic experts, who apply the AI-collected insights to help completely and accurately assess patient language, word choice and more. The level of feedback available via AI and coupled with expert analysis helps break down obstacles to patient study success, design studies that are responsive to patient insights, and reduce costly participant drop-outs in longer-term studies—ultimately addressing the two greatest challenges facing the industry: clinical trial recruitment and retention.
2. Augmenting Study Teams Via Predictive Analytics
The more data that is available, the more helpful automation will be. In decentralized clinical trials (DCTs), research study sponsors use a wide array of tools to collect data from participants remotely. Not only does this help reduce the need for participants to visit clinical trial sites—an important key to making research accessible for more people—but they also help with collecting more data than would be possible in traditionally designed research studies.
Many tools, such as eCOA, eDiaries, wearable sensors and mobile medical devices (e.g., spirometers, pulse oximeters and even mobile ECG) allow you to collect data more frequently and, in some cases, continuously. This vast amount of data benefits from the use of advanced AI and ML to quickly glean insights that can help study teams. For instance, machine-learning algorithms can be used to detect specific markers in the data that can predict study dropout risk. By informing study teams with insights on specific patients who are predicted to be moving toward dropout, early steps can be taken to provide the support that patients need to remain enrolled in the study.
3. Performance Benchmarking
The use of AI and machine learning to identify trends and insights that help make studies more efficient and effective is steadily expanding. Using real data across hundreds of clinical studies, researchers are identifying the specific factors that characterize highly successful trials, as well as those indicative of trial challenges.
Although still early in this process, clinicians are using AI and ML to learn from data insights, as opposed to relying merely on ad hoc feedback from study engagement. The ability to provide a "Trip Advisor-like score” to assessments, features and users is helping researchers to compare data and develop new data baselines to benchmark performance. (Full disclosure: My company provides this type of solution, as do others.) Employing machine-learning algorithms to update these scores and provide visibility to clinicians can help them easily monitor how their study is going and quickly react if the score begins to drop.
4. Shifting Manual Data Entry To Automated Data Entry
Data entry is time-consuming, and for a team of highly trained and talented professionals, it’s not the most productive use of time. AI and/or ML can help study teams focus on higher-value tasks. In clinical research, this means spending more time engaging with participants and less time using technology tools.
One such advantage is entering data one time in one system that then updates all other systems and fields via an automation with an audit trail. Pulling data from one system and populating other systems to drive the highest-quality data occurs today, but it has extensive room for improvement using new technology tools. Our industry is in the middle of this shift now and has not arrived at our preferred destination yet, but researchers can be encouraged with the early progress to date.
With so much data available and so much at stake—the successful development of new therapies—the time has come to begin engaging these AI/ML use cases to better listen to and gain insights from more patients and to make clinical research more efficient. Automation through AI and ML can help, and these and other use cases should encourage the entire industry to start on this journey today.